White paper – BMS
Embedded digital twin technology for BMS
Leveraging physics-based embedded digital twin technology
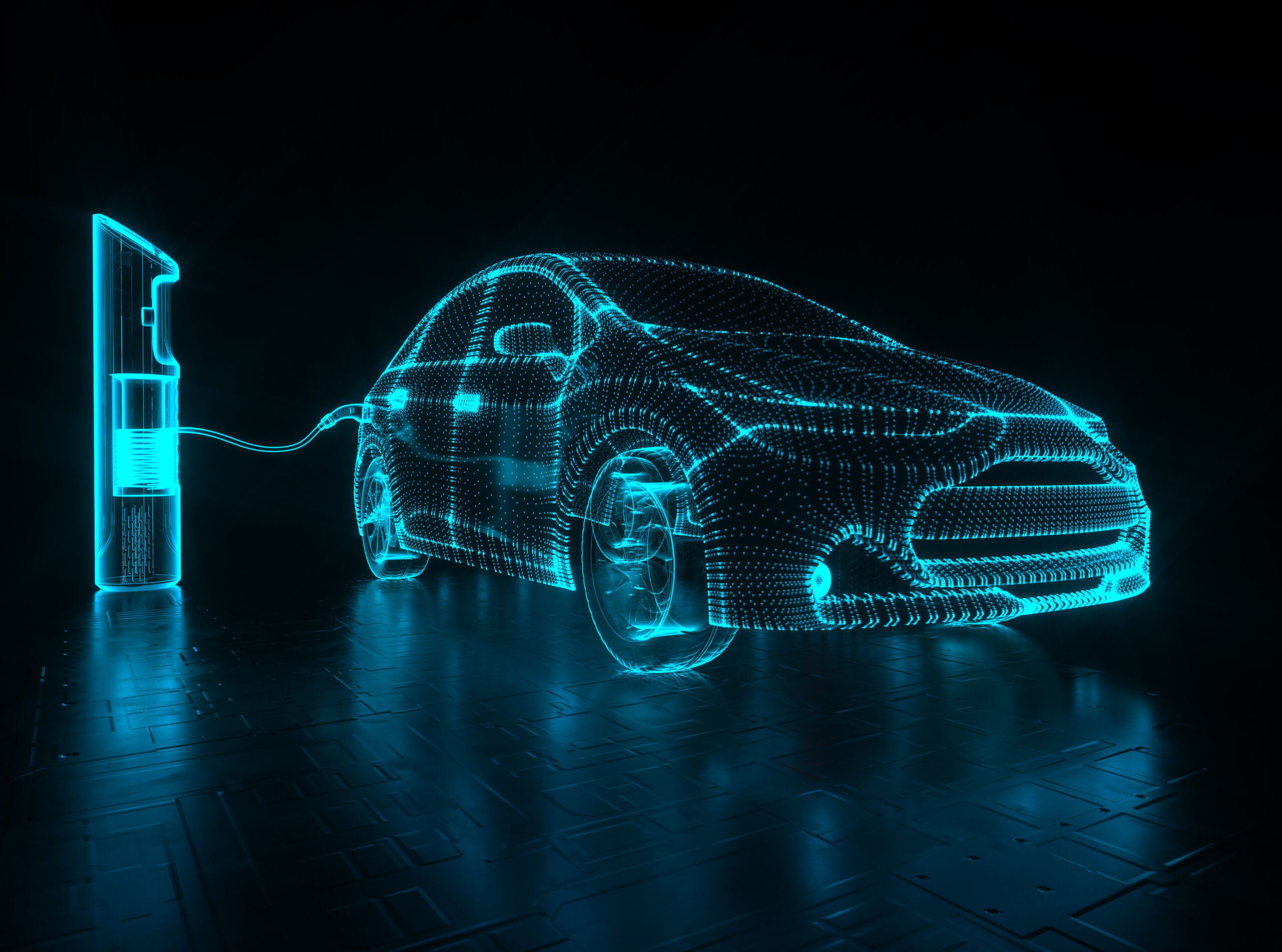
Embedded digital twin technology– Executive summary
Current state-of-the-art battery management systems (BMS) incorporate battery models to maintain batteries in a safe condition and estimate the state of charge (SOC) and state of health (SOH). However, a new idea of next-generation BMSs can be significantly improved by enabling State-of-Function (SOF) optimization, i.e. the available power limits calculated to maximize the battery’s power output and, at the same time, its lifetime. The real causes of aging are the electrochemical and mechanical processes occurring within the cell. Therefore, BMSs that integrate physics-based modeling of the most severe degradation mechanisms in a reasonable manner (e.g. plating, formation, and growth of the solid-electrolyte interface), allow for the implementation of control strategies that significantly improve the overall system. In addition to introducing performance advantages,leverage the physics-based BMSs can redefine the paradigms of operational safety and lifespan, as well as positively re-evaluate the second life of energy storage systems (ESS) in the case of automotive batteries.
This paper aims not only to expose the obvious technical advantages introduced but also to highlight how the industry-wide adoption of the next generation of BMSs will bring enormous economic benefits for both ESS manufacturers and users, providing a key tool for achieving the goals set by the European Green Deal in terms of diversification of supply sources and optimization of battery raw materials, to finally achieve strategic autonomy in one of the most fundamental sectors of the future green and digital economy.
Embedded digital twin technology– State of the art
Energy storage systems are a key factor in the energy transition currently taking place worldwide. The growing demand for electric vehicles in the transport industry and for renewable energy sources in the power generation sector has led to the need for increasingly efficient ESSs. One of the most promising types of energy storage is provided by lithium-ion batteries, as their high power and energy densities meet the requirements of a wide variety of applications.
The cost of lithium-ion batteries and the limited raw material resources calls for the maximization of battery life. In addition, it is crucial to optimize the power and available energy capacity of batteries by minimizing the irreversible cell aging phenomena, rather than simply oversizing
the ESS as a cauthelative safe margin against quick aging.
To meet these objectives, battery management systems must be improved. BMSs run battery models to keep the battery in a safe condition and to be able to estimate the state of charge, state of health, and state-of-function, (a calculation of the limits on the available power that seeks to maximize battery output while maximizing battery life). BMSs commonly use heuristic algorithms that keep the cell within predefined safe working points or empirically based phenomenological models, such as equivalent circuit models (ECMs), which can provide accurate estimates of battery SOC. However, these models do not describe the internal electrochemical physical variables of the battery, so it is impossible to use them to estimate the battery’s internal functioning, which is necessary to predict aging phenomena. Therefore, the use of empirical models leads to a poor prediction of SOF.
Other recent attempts exploit artificial intelligence (AI) techniques to estimate SOH and residual useful life (RUL). The capabilities of these algorithms are highly dependent on the data available for the training process. Although this has proven to be a powerful tool for studying the effect of aging in state estimators, if it is necessary to calculate internal states, physics-based models (PBM) are crucial.
All these considerations highlight the necessity of using the PBMs of lithium-ion cells in the BMSs of ESSs. These models are based on equations developed from first-principles physics that describe the internal physical behavior of the battery cells.
With the improved understanding that PBMs can provide, the BMSs can be programmed to accurately predict the state of the aging mechanisms occurring within the battery cells and control the battery based on these predictions. This allows the actual energy and power capacity of the batteries to be determined accurately and in real-time, avoiding oversizing. In addition, the use of these models can ensure optimized battery life management to avoid undesirable aging effects.
The combination of PBMs and AI has recently emerged as a promising field of research. In this context, reduced-order models (ROMs) can be used to efficiently feed AI algorithms or to provide feedback to these same algorithms while they are running in the BMS. Furthermore, AI techniques can be used to help manage uncertainty in predictions or model parameters. Taking all these considerations into account, a clear research gap can be identified: the challenge of efficiently implementing PBMs embedded in BMSs. Exploiting PBMs to enable accurate SOF determination, either alone or in combination with artificial intelligence algorithms, is another key challenge.
Last but not least, the experimental validation of these models is crucial, as proof of their validity in real scenarios is needed to definitively validate the concept. Overcoming these challenges will lead to more efficient use and longer life of lithium-ion batteries.
Embedded digital twin technology– Next generation of BMSs
Today, the SOC of the cells is well-defined, and established methods can be used to calculate accurate estimates of the state of charge and to provide confidence intervals on the estimates. Similarly, there are validated methods for estimating cell resistance and total capacity and obtaining SOH estimates. The calculation of cell energy is simple, and different types of cell balancing strategies can be implemented, with different levels of complexity and speed. All these aspects can still be improved, but the current state of the art offers adequate battery management for many applications. The long-term effects of such existing methods on battery packs is something that still needs to be proved, simply because there are currently few examples of high-capacity lithium-ion battery packs that have been in use for a decade or more.
The most significant improvements that can be made to battery management methods concerning how power limit calculations are performed and some of these new ideas will be introduced in this section.
A BMS that incorporates state-of-the-art methods works fine in general, but in many cases, the battery pack will also result oversized. The problem lies in the way power limits are currently calculated. The basic requirement imposed on the calculation of these limits is that certain maximum and minimum voltage limits must never be violated by any cell in the battery pack. But why? The real concern is cell degradation.
The basic assumptions are that:
• If voltage limits are violated, then the cell will degrade quickly and fail prematurely.
• If voltage limits are properly maintained, the cell will have a long and productive life.
Safety guarantee is the main concern of a BMS. However, cell terminal voltage alone is neither the root cause nor a good indicator of most safety hazards. Rather, hazards that are latent during normal cell operation can become serious risks when certain cell degradation mechanisms are triggered. For example, overcharging a lithium-ion cell can cause metallic lithium plating, which forms lithium dendrites that can short-circuit the cell and lead to thermal runaway. These mechanisms are related for example to the local internal anode potential vs Li/Li+ reference electrode, which is different from the cell voltage. The cell voltage is a non-linear combination of several electrochemical quantities, and its measurement hides the whole local effects.
If you model degradation mechanisms at critical points within the cell, you can also avoid the corresponding security risks.
From this discussion one very important lesson is learned: the real causes of aging are the electrochemical and mechanical processes occurring within the cell, and the cell’s terminal voltage is just a poor but easily measurable indicator of the state of these processes. Statistically speaking, based on numerous empirical cell tests, the manufacturer has established that the cell offers a reasonable compromise between performance and durability if a certain voltage limit does not exceed. However, under some conditions it is perfectly fine to exceed this value; under other conditions, even if this value is maintained, unacceptably rapid aging may occur, especially for older cells whose internal dynamics have changed. Therefore, the real issue in calculating cell power limits is not the terminal voltage of the cell, but rather the amount of aging or degradation expected to occur at different power levels.
Cell power limits should be calculated to optimize a trade-off more directly between the performance provided by the cell and the incremental degradation rate experienced by
the cell. To do this, it is necessary to mathematically model the degradation and devise optimized model-based controls to calculate the best trade-off.
Some works in literature have suggested that, if this is done correctly, battery packs in some applications might be able to deliver more than 200% of their voltage-limited power without compromising durability. Alternatively, the battery pack could be significantly reduced in size and still provide the required performance.
These possible cost savings and/or performance gains are sufficient incentives to make a strong attempt to design power limits based on cell physics instead of the terminal voltage. The interactions between the ideal cell behavior and different degradation mechanisms are complex. In addition, most cell manufacturers include chemical additive packages that are part of the cell construction and that do not participate in the operations of the ideal cell but are instead designed to prevent degradation. These additives are trade secrets and therefore not disclosed to the BMS designer. This makes accurate modeling of degradation rates very difficult. However, it is worth considering that it is not mandatory to perfectly model all mechanisms to obtain a useful result.
Furthermore, to control cells by means of physics-based power limit calculations, it is not needed to model any mechanism that is not influenced by a variable we influence over. In the hypothesis of good degradation models, there is the possibility of designing controls that make a significant difference even if some minor effects are neglected.
As already mentioned, one of these models is the solid–electrolyte interphase (SEI) formation and growth. This degradation mechanism is believed to be the most significant life-limiting mechanism in cells having graphite-negative electrodes.
So, if cell controls that slow down SEI growth, are adopted, then the battery life can considerably be extended. Another model is the one of lithium plating on overcharge.
This mechanism would not normally occur if the manufacturer-specified upper voltage limit on the cell is preserved, but it is something that needs to be monitored carefully since it could bring cell failure in a few cycles if triggered.
It is worth noting also that the plating effect can occur even if we respect the manufacturer-specified upper voltage, for example when there are low temperatures. Thus, we must model lithium plating and take it into account when computing cell maximum power limits.
All the above leads to the conclusion that the implementation of physics-based models directly into the control of the BMS to estimate the internal electrochemical states of the battery to model degradation mechanisms and adjust power limits taking these phenomena into account is the best way to improve the state of the art in the industry.
However, the main bottleneck in implementing PBMs in BMSs is that they have a much higher computational complexity than empirical models and therefore require extremely powerful computational resources, if at all feasible. To overcome this drawback, model order reduction methods can be applied by creating physics-based ROMs. ROMs are computationally simpler models that can highly accurately predict the same internal physical variables. These ROMs can be run in practical BMSs and thus battery controls can be designed based on these estimates of physical variables.
NEWTWEN develops the technology required to address the following challenges related to a new generation of BMSs capable of significantly impacting the market:
Implementation of physics-based models of the entire embedded hardware controlling the battery pack.
Identification of PBMs parameters.
Modeling of the main cell degradation phenomena.
Implementation of optimal controllers to maximize the state of function, i.e., calculate power limits while maximizing battery performance and lifetime.
Experimental validation.
The software libraries developed by NEWTWEN to automatically generate so-called embedded digital twins of battery packs can reduce PBM models to such an extent that ROMs can break the bottleneck of real-time implementations on standard microcontrollers with limited computing power and memory.
Experimental validation is crucial, since the validity of any model, particularly in this context, must be proven through real-world operating profiles.
Considering that, it is worth noting that estimates of most battery states such as SOC or SOH are not experimentally validated, as there is no sensor measuring them directly. Few certify the accuracy of these estimates, and even fewer, if any, guarantee the accuracy of SOH estimates.
On the other hand, the physics-based approach proposed for the new generation of BMSs can be experimentally validated since the phenomena of solid-electrolyte interface growth and plating can be measured by, e.g., opening the cell and verifying whether and in what quantity these phenomena have occurred, thus having a so-called ‘ground truth’ to compare with the mathematical models that predicted the estimate. This greatly enhances the approach, making it increasingly promising to become the new state-of-the-art in the industry in the coming years. (Figure 1)
Embedded digital twin technology – Benefits
It has been seen that none of the cell degradation mechanisms are directly related to the terminal voltage of the cell but are rather a function of the internal stress factors of the cell.
Therefore, assuming that the degradation mechanisms can be well modeled, it makes more sense to calculate power limits based on the expected capacity loss and/or impedance increase due to these stress factors, rather than calculating them based on voltage limits.
There is much work to be done before the proposed BMS control solution becomes generally practical, but the potential benefits indicate that this effort is worthwhile.
In this section, it is shown how physics-based degradation mechanisms for calculating better power limits lead to significant economic benefits. In particular, the following control problems can be considered:
1. For applications such as EV, E-REV, or PHEV where the battery pack is charged from an external source: What is the optimal charging profile? Is fast charging possible? For a fixed charge duration, what is the best charging strategy?
2. For any dynamic application, including all xEV applications while driving a car: What is the maximum absolute charging power that can be maintained in the following ΔT seconds? What is the maximum discharge power that can be maintained in the following ΔT seconds?
3. Considering xEVs as storage units for the smart grid, when does it make sense to lend energy to the grid? What should the rental rate be to allow energy lending?
For these issues, different types of optimized controls might be more suitable.
The fast-charging case is considered, in which the cell initially has a specified SOC value between 10% and 90% and the charger must optimally charge the cell from this initial state to a SOC of 90% in a specified period of two hours. The SOC of the cell cannot fall outside the range between 10% and 90%, while the current is considered soft-constrained.
Also, SEI and plating degradation models together are considered, as well as the thermal evolution of the battery pack to avoid overheating the cells. At this point, to calculate the best charging strategy, a constrained optimization problem must be solved by minimizing the objective function as a function of the current that achieves the desired SOC, considering the constraints imposed on SEI, plating, and limiting temperatures. Once the current references have been calculated, the power limits are then calculated using the imposed voltage limits. The results in (Figures 2-3) may be surprising.
The optimal charging strategy, using this BMS, is to rapidly discharge the cell to the minimum allowable SOC, wait as long as possible (due to SEI minimal growth) and then charge the cell not too quickly (due to constraints on the SEI growth and plating phenomena) to the desired final SOC. The cost of discharging and charging proves to be lower than the cost of maintaining a high SOC for an extended period.
Thus, it can be estimated a comparison over time between the industrial state-of-the-art BMS that implements equivalent circuit models ECMs, calculates power limits based on voltage/current (CC-CV), and estimates SOC and SOH, with the new generation of BMS that implements PBM models, calculates power limits based on degradation models, and estimates SOC, SOH, and SOF.
Therefore, until now it has been shown, how using a new generation and concept of BMSs, it is possible to control batteries while better preserving their residual life, as shown in Figure 4. Now, it is considered how this can be translated in terms of value.
The SOH of an electric car battery is a key factor in determining the health of the battery and its safety under operating conditions, but it is also a very important parameter in assessing the residual value (RV) of the electric vehicle.
SOH can affect the cost of the battery by up to 55% of the total cost of the vehicle, which means that by decreasing its decay of it, the depreciation of the battery in the market can be reduced. Four examples of monetization related to the SOH parameter are shown in the following.
Example 1 (DEPRECIATION)
Considering that the average depreciation of an electric car is 52% in the third year of ownership, which is still higher than the depreciation of the internal combustion engine (ICE), according to some still limited studies in the field, there is a strong correlation between SOH and the entire RV of the electric car. It can be assumed that for every 1% increase in SOH, to date, the whole RV of the electric car will benefit by + 0.5%. This means that if considering the trade of an electric car after 3 years of adopting a standard BMS and comparing it to the same car implementing a new generation physics-based BMS, it is possible to determine an increase in the RV of up to + 2.25%, which means that the devaluation of the electric car will be reduced to 50.83% over the 3 years. This may seem insignificant but considering that the average price of an electric vehicle in the EU is €43720, it means a difference of €512 per vehicle sold. The financial impact of this effect on the overall electric vehicle market is easy to understand if the expectations for the coming years of electric vehicle market penetration are taken into account.
Considering only 100,000 electric vehicles sold, this means a total value of approximately EUR 50 million. It is worth noting that this value means both a strong decrease in the total cost of ownership (TCO) of the electric vehicle from the customer’s point of view, which makes electric cars more attractive, and a huge opportunity for the second-hand market.
Example 2 (SECOND LIFE)
Today, the price of lithium batteries for stationary use is equal to that of batteries produced for electric vehicles. Considering that the average installed capacity for the latter is far higher than that required for stationary use, the price could be even higher. Looking ahead, when batteries are exhausted according to the automotive sector criteria, i.e., reach a SOH of 80% of their initial nominal value, the price of second-use batteries for stationary applications will be rationally lower. The price will depend on various factors, but will probably depend on the alternatives that the market will present, such as:
- Buying new batteries from the primary automotive market but manufactured for specific stationary purposes. (Upper limit)
- The cost/opportunity provided by alternatives such as recycling (lower bound)
- The actual cost of remanufacturing is required to obtain new mechanical and control components for specific stationary use.
Making some assumptions about the current market price, the value for each percentage point of SOH saved for second-life application can be estimated to be around € 255.
Example 3 (WARRANTY AND ACCRUALS)
In general, any company may have a warranty policy whereby it promises to repair or replace certain types of damage to its products within a certain number of days from the date of sale. This is certainly in the automotive sector, where a warranty network covers new gas-powered cars, and electric vehicles have similar coverage. Typically, a warranty covers all components of a new car for a certain period or for the distance that is reached first (e.g., 36,000 miles in ICE-powered cars). xEVs have the same warranties, plus an additional, specific warranty for the battery, electric motor, and other electrical parts that are unique to xEVs. Warranties on electric powertrains can vary from manufacturer to manufacturer, but also from region to region. Typically, they cover at least five years or 60,000 miles, although some last up to 10 years or 100,000 miles:
- In the EU, industry standards for automotive battery warranties are set at 160,000 km or eight years, whichever comes first.
- Warranty coverage for hybrid and electric vehicle batteries is set by US federal law, which requires car manufacturers to warranty electric and hybrid vehicle batteries for at least eight years or 100,000 miles (California goes further, requiring a 10-year, 150,000-mile warranty on electric and hybrid vehicle batteries).
This means that if the company is able to reasonably estimate the number of warranty claims that might arise under the policy, it must set aside an expense to reflect the cost of such anticipated claims, and this provision must be made in the same reporting period in which sales of related products are recorded.
As far as new electric powertrains and batteries are concerned, there is not yet sufficient data to reasonably estimate the number of warranty claims that might occur over a certain period.
This makes it extremely difficult to estimate the amount to be set aside for each vehicle to cover warranty claims arising from failures/ premature aging of electric powertrain components, resulting in a loss of performance.
Adopting a physics-based BMS for this type of application would allow to:
1. Obtain a positive net return from the absence of warranty claims over the period considered, due to the improvement in battery health decay. Considering an average 2.8% rate of accrual in the automotive world in 2021, on components that have been worked on for years, this would mean a potential increase in sales of around €280 per car. This, considering the number of electric vehicles sold per year of only 100,000 units, would mean a financial impact of up to
€ 28 million.
2. Calculate an appropriate installment amount without risking a low annual return. The OEM could decide to decrease the accrual amount, considering the lower estimated expenses for warranty claims, being able to sell their products at a lower price, and further improving the TCO of the vehicle (Figure 5-6).
Example 4 (SUPPLY CHAIN AUTONOMY)
The expansion of the battery industry will be crucial to meet the growing European demand for batteries. This is in turn driven by the rise of electric mobility, which reached new heights in 2021, when, despite the coronavirus pandemic and significant supply disruptions, Europe continued its efforts and managed to consolidate its leading position in the global market for sustainable batteries. To date, 111 industrial battery projects have been developed in the EU Member States, with around 20 Gigafactories of battery cells. The EU is set to meet 89% of its growing battery demand by 2030 and should be able to produce batteries for up to 11 million cars per year, an estimated 600 GWh per year in terms of batteries. Also, to be considered are the electric cars registered between 2020 and 2022, estimated at about 3.5 million, for which the new regulatory framework on New Rules for Battery Production, Recycling, and Reuse will have to be met. A general increase in the SOH level of batteries in the market would in turn lead to:
- A longer lifespan for lithium-ion automotive batteries, which means that new batteries produced in the EU could be used in new electric cars, without having to reduce the industrial production of EU car manufacturers that rely on EU-produced batteries.
- Easier achievement of the collection targets for spent car batteries, which for light-duty vehicle batteries will be 51% in 2028 and 61% in 2031. All collected batteries must be recycled and high levels of recovery must be achieved, especially for valuable materials such as copper, cobalt, lithium, nickel, etc. Embedded digital twin technology. Embedded digital twin technology. Embedded digital twin technology. Embedded digital twin technology.
Embedded digital twin technology – What are the next steps?
Experimental validation for the proposed new generation of industrial BMSs based on physical cell models is crucial. As already mentioned, the proposed approach can be experimentally validated. To this end, NEWTWEN is establishing strong strategic partnerships with major industry players to demonstrate the added value in a concrete way.
The first barrier for real-time implementation has been overcome thanks to NEWTWEN’s software libraries for model reduction and the technological advancement of the microcontrollers used in the specific application. Challenges remain regarding the validation of the degradation models and the demonstration of the potential added value in economic terms.
The direction taken is to forge partnerships with specialized companies and laboratories to effectively demonstrate both quantitatively and qualitatively the estimation of physical phenomena such as SEI and plating, quantifying the life extension achievable using the next-generation BMS compared to the most advanced state-of-the-art industrial technology. Furthermore, the objective will be to certify the activities and results obtained in order to gain confidence and establish new requirements for the quality of battery testing.
https://www.newtwen.com/aboutus
https://www.linkedin.com/company/newtwen/